Remote Sensing
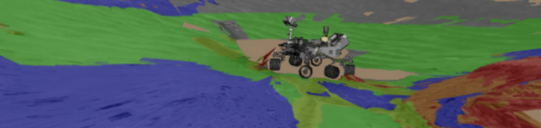
Remote sensing is the measurement of radiation from objects not in immediate proximity to the observer, using sensors on satellites or aircraft to analyze physical, chemical, and biological properties of the target objects. It's used to study the Earth's surface and other planets, and machine learning algorithms can pre-sort the data to extract useful information. In-situ experiments validate the data and provide more information. This comprehensive approach enables discovery of new insights and knowledge about the natural world, supporting scientific, commercial, and policy applications.
Weakly supervised segmentation is a subfield of remote sensing that uses machine learning algorithms to perform image segmentation with limited annotation. The approach is based on the assumption that some weak labels, such as image-level annotations, are available for training. This approach is useful when the cost of obtaining pixel-level annotations is high, and when the dataset is large and diverse. In weakly supervised segmentation, the goal is to train a model that can accurately segment the target object by leveraging the available weak annotations. Despite the challenges involved, weakly supervised segmentation has shown promise in various remote sensing applications, including land cover mapping and urban area detection.